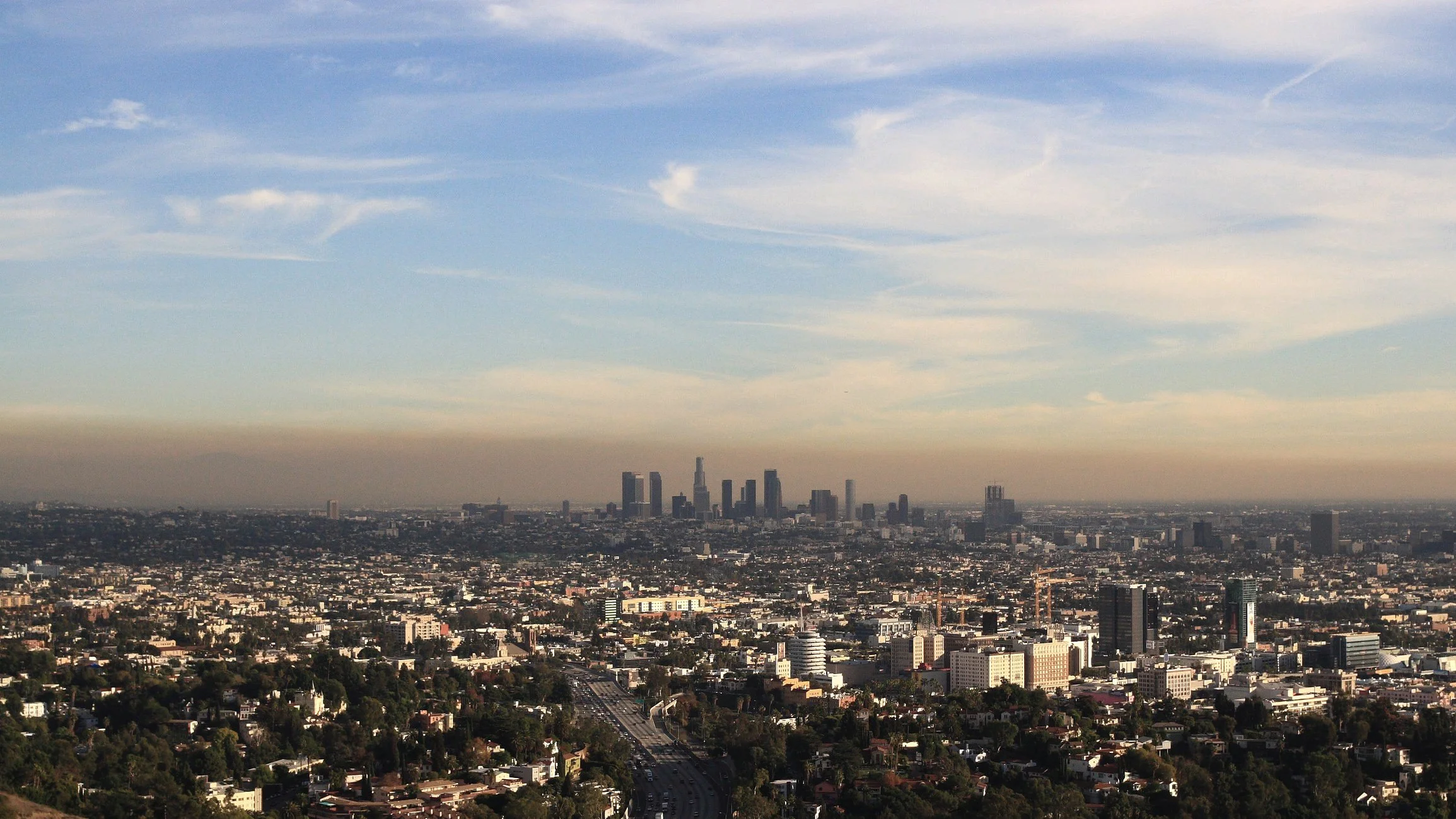
Research (under construction 🚧)
Summary of past and present research projects.
in-situ ice particle images from a Cloud Particle Imager (CPI)
ML models trained to predict 3-D features from 2-D images
Synthetic ice crystal proxies that were computationally generated
A Machine Learning Framework for Predicting 3-D Attributes of Ice Crystals from Cloud Particle Imagery
Status: in progress
Summary: Clouds exert a huge influence on Earth's climate and water cycle. However they contribute to some of the largest uncertainties in climate and weather models. Ice clouds are composed of many tiny ice crystals with diverse habits (i.e., shapes). Ice habit impacts microphysical processes, as well as cloud-radiation interactions. 2-D images of ice cloud particles are available from in-situ measurements, but we would ideally like to characterize 3-D properties of ice crystals to constrain the mass-size relationships used downstream in atmospheric models. In this work, we present a machine learning framework to predict 3-D features from single 2-D images, using synthetically generated crystal proxies as training data. We find that machine learning outperforms naive linear regression, and can predict 3-D features such as effective density and effective surface area with high accuracy.
Investigating the Impacts of Anthropogenic Heat on the Climate of Los Angeles
Status: in prep
Summary:
Anthropogenic heat (AH) is waste heat resulting from human activities. AH results from energy consumption, primarily from traffic, buildings, and human metabolism. In this study, we aim to (1) quantify the magnitude and spatio-temporal variability of AH in Los Angeles, and (2) model the meteorological impacts of AH on Los Angeles' urban climate. To do this, we synthesized a high-resolution (100 m, hourly) AH flux dataset from disparate data sources, and then conducted sensitivity tests using the Weather Research & Forecasting Model (WRF) to evaluate the impact of AH on temperature, wind, and boundary layer height in Los Angeles.
Measuring the impacts of a real-world neighborhood-scale cool pavement deployment on albedo and temperatures in Los Angeles
Status: Published, Mar 2022 (click here for paper)
Summary:
The urban heat island (UHI) effect often causes urban areas to be warmer than surrounding rural areas. In the context of increasing global urbanization, and rising average air temperatures due to climate change, there is a pressing need for effective urban heat mitigation/adapation strategies. Our study took real world measurements to quantify the efficacy of one proposed mitigation strategy - solar refletive cool pavements. We found that a neighborhood-scale deployment of cool pavements reduced air temperatures by up to 0.2 °C and surface temperatures by up to 5 °C. However, the albedo (i.e., reflectivity) of the cool pavement was degraded by ~30% after 1 year of real-world conditions.
Measurements to determine the mixing state of black carbon emitted from the 2017–2018 California wildfires and urban Los Angeles
Status: Published, Dec 2020 (click here for paper)
Summary:
Black carbon (BC) aerosols are tiny particles that result from incomplete combustion, and BC is the second strongest warming pollutant in our atmosphere after CO2. However, there are significant uncertainties associated with the precise degree of warming caused by BC. One factor that contributes to its this uncertainty is its internal mixing state (i.e., how thickly coated is it and what is it coated with?). In this study, we conducted multiple field measurements to understand how BC internal mixing state varies as a function of source (e.g., wildfires vs. urban) and aging timescale (e.g., fresh vs. aged emissions). Our study confirmed what has been reported in a limited number of previous studies: BC from biomass burning emissions (e.g., wildfires) are more thickly coated than BC from urban emissions, and BC coating is enhanced during atmospheric transport.